In association with Elastic
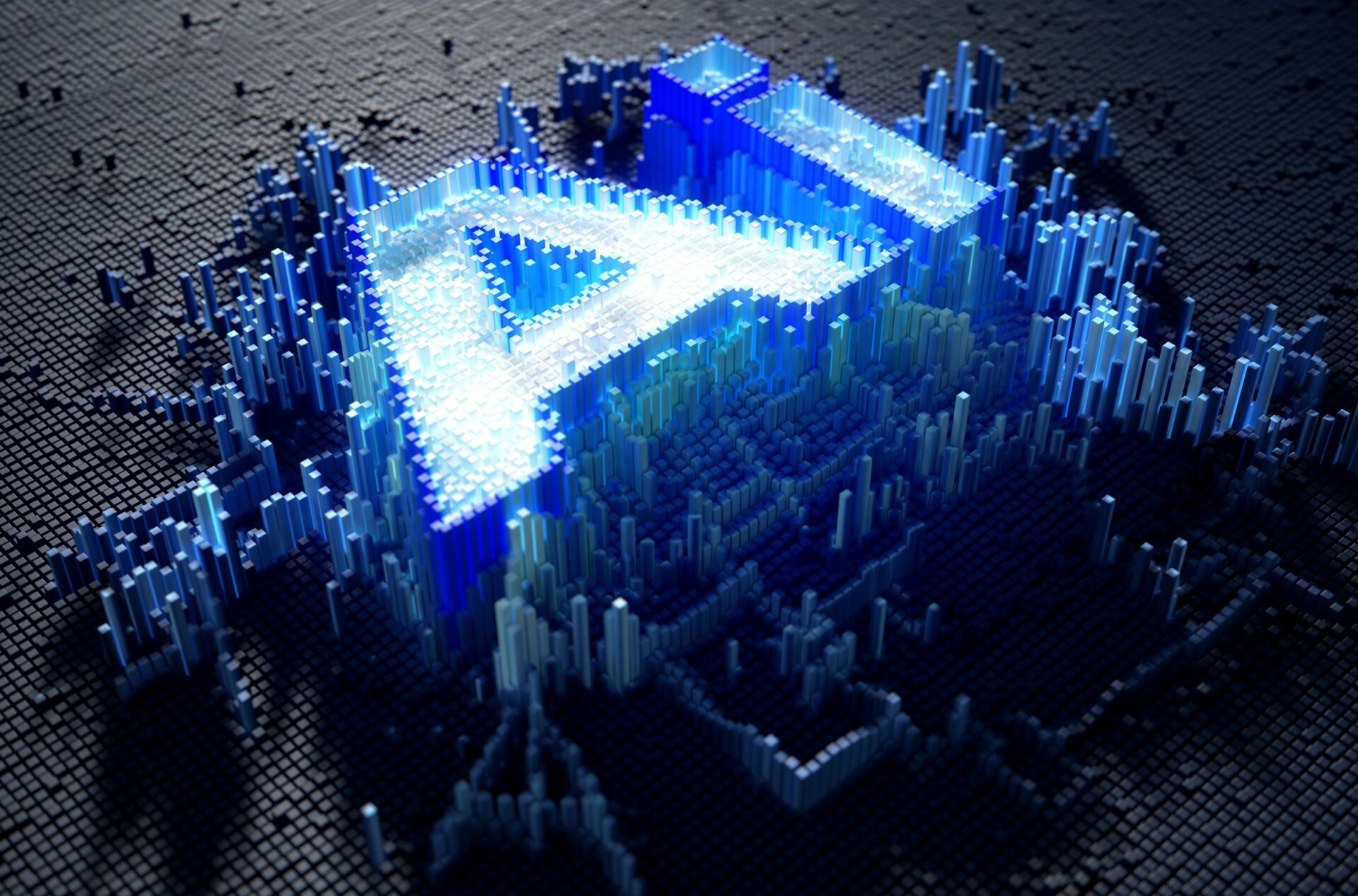
Many organisations have embarked on AI trials in recent months, driven by the urgency to innovate rapidly. However, several of them have encountered a sobering reality—the inherent complexity of implementing AI effectively.
Numerous companies embark on AI efforts believing that a simple solution can be deployed quickly. However, it can be difficult to successfully leverage AI without first having the foundational data sources in place to create a customised solution that truly works.
“You could set up a quick demo and get a solution running out of the box, but it would be a lot harder to get it tailored to your needs to deliver real results later,” said Ken Exner, chief product officer at Elastic, a Search AI company which helps businesses use data to bolster security, analytics, and AI.
He was speaking at ElasticON Singapore, which brought together more than a thousand developers, architects, DevOps engineers, security analysts, and other IT professionals to learn about Search AI and the capabilities it enables, from observability and security to enabling the development of AI.
“In a rush to get out a working demo, businesses often find it hard to customise and cannot meet more complex requirements later,” he noted.
At the same time, a lack of options to pull from different data sources means that results cannot be improved over time, so users are stuck with the same solution that quickly becomes inadequate, he added.
Investing in data and search
To get AI right, many businesses realise that they have to invest in getting their data in order first since that will form the foundation of their efforts. Without this key building block in place, it is difficult to achieve accuracy and improve responses from AI.
The rampant growth of data – from logged performance metrics, security reports, to customer profiles – means that an organisation needs to draw insights from the data in real time to identify anomalies in security and performance, or to inform what customer pain points their next product should address.
Organisations often face uncertainty regarding whether their applications are properly connected to the necessary systems. Even when they are, obtaining the correct information remains a challenge.
Unsurprisingly, in an Elastic study of 3,200 technology leaders last year, a high 89 per cent reported that their use of generative AI was being slowed.
Many now understand that one big problem is a lack of good data. Equally important, however, is the presence of unrealistic expectations.
“A typical Google search gives multiple results that people can sift through,” said Exner, “but an LLM (large language model) chatbot pushes one poor answer to you if it doesn’t have good data, resulting in poor responses.”
“The key to getting your generative AI applications to succeed is getting the right data the application needs at the right time,” he added.
Find the best way forward
To make AI efforts successful, Elastic makes obtaining the right data quicker and more accessible with its hybrid search comprising text, semantic and vector search.
The company’s implementation of advanced search capabilities like vector search and retrieval-augmented generation (RAG) is critical for advanced generative AI applications. Its RAG model enhances the accuracy and personalisation of AI responses by connecting the latest, most up-to-date facts and relevant information.
Businesses should also tune results for relevance, advised Exner. Conduct A-B testing to evaluate models, for example, hybrid versus vector search, before sending an LLM to production, he added.
He said Elastic can help businesses get their data to build a strong foundation in preparation for their big AI push.
The company currently supports 400 connectors and integrations to data sources, so it becomes easier to build up a data lake for the AI to learn from, he pointed out.
Elastic has separated compute and storage in its data lake architecture, where it has decoupled things like indexing from search and scale.
This way, Elastic is able to work with existing systems that customers already have in place and then put the data that is necessary in its data lake. A customer thus has the choice to choose and work with the data sources they have or manage the data with Elastic.
To get started, businesses can try out the Elastic Search AI platform, which ingests and optimises all data types, from any source. Once ingested, the data can be holistically searched, analysed, and acted on in real time.
Driven by Search AI, the platform delivers the speed, scale, and relevance needed for any data-centric use case – from building custom search experiences, to monitoring apps and infrastructure, to modernising security operations.
It is available to businesses in several options, including one that is serverless and fully managed. Businesses start with as little cost as they wish, to see if Elasticsearch helps to build up their AI. Those who want more control can opt for a self-managed version or a hosted version.
Looking ahead, Exner said businesses should see better results with AI soon, as many become more focused on their AI efforts.
For example, using RAG techniques to provide context for LLMs will help chatbots deliver better, more relevant results. This also beats building one’s own models from scratch.
Using RAG means businesses can control what information to deliver over generative AI, producing more accurate results and ensuring that only users with the right permissions can access sensitive information.
Exner gave the example of a chatbot that answers questions on a company’s human resources. Only those with the proper credentials would be able to find out how much the CEO earns, for example.
With RAG, such access can be contextualised and customised, based on the organisation’s own data, said Exner. This is a much more efficient way than trying to build individual models to cater to so many different use cases in an organisation, he added.
Find out how Elastic can boost your organisation’s AI efforts here.