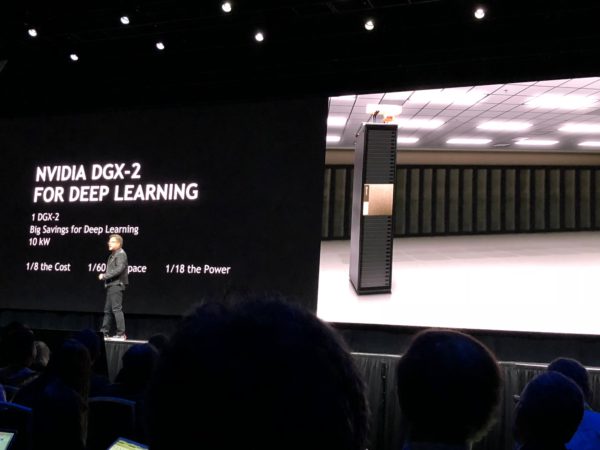
Nvidia’s GPU Technology Conference last week was as techie as a scientific conference.
Walking into the San Jose McEnery Convention Center, you find rows of poster exhibits. Hundreds of posters on significant innovations or improvement using GPU computing were exhibited in the hallways.
More than 8,000 attendees were at the four-day GPU tech fest where they attended over 600 sessions on topics like self-driving cars, high performance computing, smart centres, computer and machine vision and virtual reality.
Mostly many wanted to hear what CEO Jensen Huang had to say about Nvidia, the company he co-founded 25 years ago. Unless you’re a PC enthusiast, you may not know a lot about Nvidia, which is headquartered in Santa Clara, California.
It makes no fancy gadget or mobile app. Instead its technology is centred around making the brawny microprocessors called GPU or graphics processing units that crunch the complex calculations necessary to make first-person shooters come to three-dimensional life on a PC.
Except that these muscular microprocessors gave Nvidia new growth opportunities, allowing it to ride the artificial intelligence (AI) wave, and stay ahead of the tech curve.
It turns out that the GPUs are well-suited for crunching tens of thousands of mathematical calculations simultaneously which makes them a standout for inferencing, simulation and visualisation tasks.
At the conference last week, the company rolled out a number of AI offerings to power simulations for robotics and autonomous vehicles as well as graphics chips for workstations and data centres.
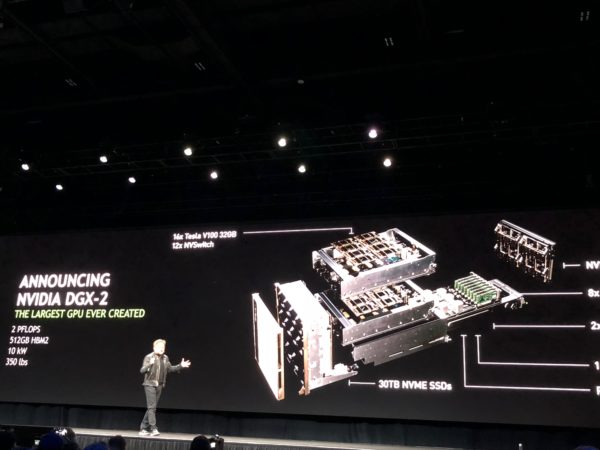
Tracy Tsai, Gartner’s research vice-president, believes that Nvidia is well-placed to ride the AI wave. She told Techgoondu that the GPU is one of three critical factors needed to accelerate AI’s work, the other two being large amount of data and a deep neural network.
“AI is the new battle ground for all technologies segments in the future. Having high performance computing and accelerators to support training and inferencing the (data) models is the must-have requirement for technologies to work,” she said.
Founded in 1993, Nvidia has quietly reinvented itself in the last 10 years while the rest of the world followed the machinations of FAANG and BAT – Facebook, Amazon, Apple, Netflix, Google and Baidu, Alibaba and Tencent.
CEO Jensen Huang, who is American and lives in Santa Clara, is behind Nvidia’s supercharged growth and what a journey it has been. For the fiscal 2018 quarter that ended on Jan 28, the company reported a record revenue of US$2.91 billion, up 34 per cent year over year.
Its highest-growth business is the data centre segment that supplies processors for AI work, which hit US$606 million, a 105 per cent growth year over year. This was the 11th consecutive quarter of sequential gains in this segment.
Speaking to journalists last week, Huang said: “In the last 10 years, we had two fundamental reinventions. First, we reinvented ourselves from (being) a PC graphics company to a parallel computing company.”
“Second, we decided on a few vertical markets that we will build solutions for, namely in high performance computing, professional graphics and autonomous vehicles,” he added.
The risks he took are paying off. While the autonomous vehicles (AV) market is in its infancy, industry observers are painting a huge market of about US$10 trillion in the coming years, said Huang.
He added that that the high performance computing market alone is in the region of US$30 billion to US$40 billion. The PC graphics market continues to be large at about US$6 billion.
Nvidia is firing all cylinders to stay ahead of the game. In the quarter ending January 2018, it coughed up US$507 million in research spending, according to Yahoo financial charts. Last year, it spent US$1.683 billion in R&D, about 30 per cent higher from US$1.05 billion spent 10 years ago.
A new product from its research is the NVSwitch, a data transfer system that enables the GPUs to communicate with each other simultaneously at a speed of 2.4TB per second.
Huang pointed out that AI researchers need much larger GPUs for tasks like deep learning. The GPU performance can be enhanced if communications between the microprocessors were fast. Nvidia research studied the problem and rolled out the NVSwitch.
“Nobody told us to develop this switch and we don’t know how big this switch market is going to be. But we saw the challenge and solved it. It is aimed at AI and high performance computing researchers and scientists.”
This switch, which is the first switch technology from Nvidia, enhances its deep learning platform to deliver a 10x performance boost on deep learning workloads compared with the previous generation six months ago.
How will NVSwitch contribute to AI? Scientists have trained deep learning neural networks based on existing data, for example, to recognise an image of a truck. The second step is to pick out a truck that the neural network has never been shown before.
This is called inferencing, or applying the learning capability to new data that has never been seen before. This would be useful in areas like autonomous vehicle research.
The company also made a major breakthrough in deep learning computing with the Nvidia DGX-2, a single server capable of delivering two petaflops of computational power.
Well-suited for high performance computing scientists, the hardware which weighs a whopping 350 pounds, has the deep learning processing power of 300 central processing unit (CPU) servers.
Though it occupies 15 racks of datacenter space, it promises to be 60 times smaller and 18 times more power efficient. No price was provided for this unit.
“I encourage you to buy GPUs, and save money,” said Huang.