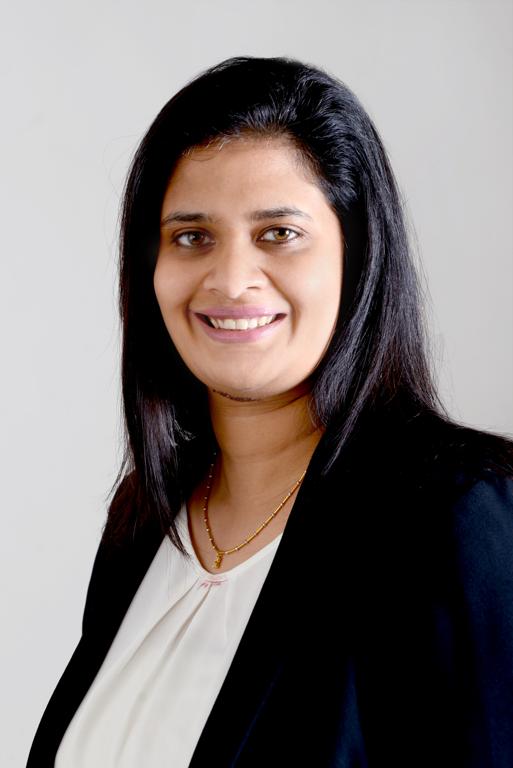
More than 36 million trade documents are processed every year at the Standard Chartered bank.
That presents a challenge not only in the volume of documents processed but also the exploding amount of data that accompany all the forms because they have to be properly documented and secured for due diligence.
Up till recently, the processing of these forms was still manual and labour intensive. This changed last year when the bank rolled out an AI-based solution which automated the processing of unstructured documents such as bills of lading insurance forms, invoices, and import and export documents.
These forms from various companies are usually not presented in a standard format hence presenting a challenge for automation. Using machine learning techniques, the AI system “learnt” the type of information in the forms and now “understands” enough to process it.
Called the Trade AI Engine, the solution has reduced the time needed to review and process paper-based trade documents by 10 per cent, said Aarthi Fernandez, global head of trade operations product at Standard Chartered.
Working with IBM, the bank fed a year’s worth of trade finance data to the AI engine where a machine learning system began to “learn” the data.
Trade finance staff were making corrections where necessary, allowing the machine to “learn” and correct itself to improve. Over time, with continuous learning, the accuracy has also improved.
Fernandez said when the AI engine was deployed early last year, accuracy was hovering about 60 per cent. “Continuous machine learning allows us to improve accuracy of the classification and defining of data elements. Our goal is to raise this to 95 per cent over time.”
Standard Chartered’s AI engine is unique with the machine learning system, she added, because there is a feedback loop. “We are using it to match data.”
AI is being examined by businesses globally for advantages in cost optimisation, personalisation of customer services and other areas. Like all IT applications, an AI solution begins with a business problem.
In Standard Chartered’s case, it needed to offer clients faster trade financing by responding speedily to submitted trade documents. “We started thinking of how to use technology to solve our business problem,” said Fernandez, who is based in Singapore.
The bank looked at various solutions including robotic processing automation (RPA) and assessed many vendors. But it decided against RPA which is a form of business process automation.
“Our data is very rich,” she explained. “RPA will work on smaller problems, we’ve 44 markets across the world, we needed a more sophisticated enterprise solution.”
After a three-month proof of concept showed the potential of the AI solution, the bank began work on the Trade AI Engine with IBM. The engine took about a year to deploy and is currently working in all the bank’s offices globally.
Fernandez said the team worked in an agile manner, in small sprints to deliver key milestones every fortnight and month. “We learnt as the project progressed, tweaked and experimented as we go along.”
She admitted there were some technical failures, but “they were stepping stones to success” adding that “when you are starting a project that no one has done before, no path has been taken, definitely there will be failures.
With the Trade AI Engine, physical inputs of data have stopped. The staff has moved from mundane work to value added work, spending quality time to analyse the data and to make sure that the due diligence checks are perfect. Faster financing for clients lead to better client servicing, she said.
Fernandez, who has spent 20 years with the bank, is extremely passionate about using technology to solve problems. Technology is the key differentiator for the bank to compete with the fintech startups.
However, technology should build skills within the organisation, she stressed. “We wanted our tech team to understand the AI technology and the architecture. They need to acquire the skills so that they will support my needs in future. And they are beginning to use the same solution in other parts of the Bank.”
Her biggest learning from this project is “to have belief and conviction in your problem and that technology can be the differentiator”.
Generally, management executives may not believe in technology because they want quick fixes for fast impact. They will get impatient, so for a project of this scale, she added, that it is necessary to get a senior leader as a champion.
For her work in this project, IBM named her one of 36 women leaders in AI for 2020. The other women leaders were from the United States, Europe, South Africa, Hong Kong and other countries.
Fantastic and futuristic solution approach to a Problem which is complex and time consuming
All the best to the team
Excellent!! Amazing effort and it is your belief & conviction that even fully paper based transaction process can be automated through digitisation AI& Machine Learning. Wish you & team under your leadership to achieve many more milestones. All the Best.